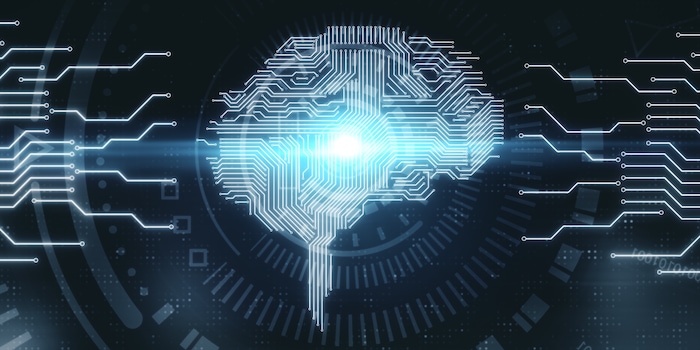
Artificial intelligence: language models form analogies like humans

The ability to think in analogies is essential for human intelligence and creativity. A trio of researchers from the University of California has investigated the ability of GPT-3 to solve new problems at the first attempt.
From solving complex problems in everyday life to creative work and scientific invention, people use the ability to draw logical conclusions from similarities. Experts also refer to this as "analogical reasoning". Cognitive psychologist and poet Keith James Holyoak, cognitive psychologist Hongjing Lu and brain and AI researcher Taylor Webb from the University of California in Los Angeles (UCLA) wanted to find out whether machines, like humans, are able to solve tasks and problems that they have never encountered before.
To this end, the researchers confronted the AI language model GPT-3, which is best known for the chat bot ChatGPT, with tasks that require it to form analogies and compared its abilities with those of human test subjects. The team found that the language model reached a level that matched or even surpassed the performance of the human test participants. This is now reported in the journal "Nature Human Behaviour".
For their test series, the researchers used the text-davinci003 variant of the Generative Pre-trained Transformer (GPT) model. Humans and machines had to conclusively complete number matrices ("matrix reasoning"), complete letter strings according to the principle of similarity ("letter string analogies") and draw literal analogical conclusions. In these three task blocks, the language model was slightly superior to the human test candidates. "GPT-3 outperformed the human subjects in the study and exhibited specifically human-like behavioural signatures across the task types," according to the article.
A total of 57 UCLA students took part in the test series for the comparison. Problems were used as a basis that neither the human test participants nor the machine could have come into contact with beforehand, as they had been specially developed for the study. When completing number and pattern matrices, GPT-3 achieved an accuracy of 80 per cent, while the human test subjects achieved an average of just under 60 per cent. When it came to completing rows of letters, humans and machines were almost on a par, with GPT-3 having a slight advantage, while the language model achieved an accuracy of around 50 per cent.
Human abilities were very broadly spread
However, the participants performed very differently from an individual perspective: while some were completely unable to solve the tasks, others achieved an accuracy of 90 per cent. However, the average value of all those tested pulled the overall result down from the approximately 90 per cent accuracy of GPT-3 to an accuracy of only 80 per cent, as 25 test participants achieved results that were in some cases well below the machine performance. In the fourth task block, in which a story was presented and the analogue story was to be selected from two similar stories, a large proportion of the students achieved perfect accuracy. The average score of all human test subjects clearly surpassed GPT-3: the AI system achieved around 70 per cent accuracy in the story block, while the average score of all tested students was more than 80 per cent. Apparently, the machine was unable to recognise the causal relationship.
The tasks were all in text form or, in the case of the number-based matrices, were introduced by a text prompt. The latter were closely modelled on the better-known progressive matrices developed by John C. Raven in 1936. This language-free type of matrix is used to measure general human intelligence, for example when it comes to categorising abstract thinking ability. Raven's Progressive Matrices (RPM) are used in classic intelligence tests for people from the age of five to old age. The test set consists of 60 multiple-choice questions in ascending levels of difficulty. Six possible additions are given for a sequence of numbers, from which the respondents can choose.
Limitations to the capabilities of GPT-3
The researchers note, however, that there are some limitations with regard to the actual capabilities of the language model: GPT-3 is not able to mimic human analogue behaviour in all areas, for example. For example, the purely text-based model lacks the physical experience in the world that enables humans to learn from accidents and mistakes and draw new conclusions. According to the researchers, another important finding was that GPT-3 is only capable of assessing analogies based on causal relationships to a limited extent. However, this is important for detecting distant similarities when comparing across stories.
The tests were also limited to processes that could be carried out within a manageable, localised time horizon. Humans, on the other hand, are able to draw on helpful sources from their long-term memory and develop new concepts based on a large number of individual analogies. Unlike humans, however, GPT-3 does not have a long-term memory for specific incidents. This limits its ability to recognise helpful similarities to an existing problem. The size of the so-called context window plays a role here: the context window is a buffer that determines the amount of text that can be processed in context. The longer the coherent text passages that a large language model can process, the longer "chains of thought" it is able to form and the deeper it could theoretically "rummage in its memory" to find suitable analogies.
Newer language models have a larger «long-term memory» than GPT-3
Newer language models sometimes have a much larger context buffer than GPT-3, which dates back to 2019. While GPT-3 can only access around 2048 tokens (i.e. around 2000 words in English and significantly fewer in German), GPT-4 already has a context buffer of 32,000 tokens (up to 32,000 words in English). The Claude model from Anthropic can access more than 100,000 tokens and the new Claude 2 is expected to be able to process 200,000 tokens in the foreseeable future without losing context, i.e. the size of entire books.
During the research period, however, the newer models were not yet available. When the researchers submitted their work to Nature in December 2022, ChatGPT had just been published and GPT-4 was still a long way off. As a result, the latest developments, which have recently gained considerable momentum, could not be taken into account. The statements made by the scientists in the article about the forgetfulness of the analysed language model should therefore be viewed with reservations at this stage. In passing, the authors of the paper touch on the problem itself and mention in a subsequently added appendix that a rudimentary test run with GPT-4 showed that this system delivered significantly better results than GPT-3. And so the research group's conclusion is clear: "Our results indicate that large language models such as GPT-3 are beginning to show the ability to find solutions to a wide range of analogy problems straight away and without prior contact points."
Spectrum of Science
We are a partner of Spektrum der Wissenschaft and want to make well-founded information more accessible to you. Follow Spektrum der Wissenschaft if you like the articles.
[[small:]]
Cover image: Shutterstock / Peshkova


Experts from science and research report on the latest findings in their fields – competent, authentic and comprehensible.