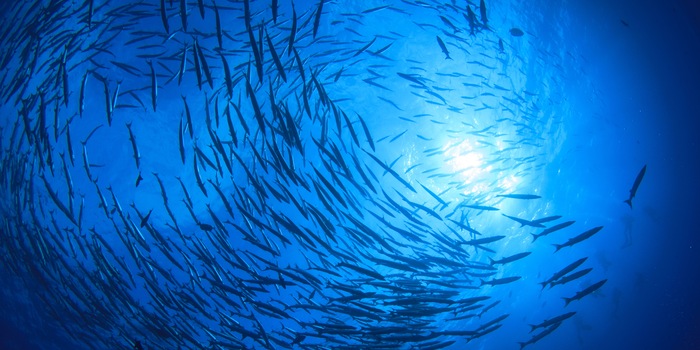
Quantum networks show swarm intelligence

Several poorly trained quantum networks together perform better than a single well-trained one. The principle can probably be applied to many typical quantum architectures.
Birds and fish do. So do bees, ants and termites. They form swarms and utilise the fact that they are stronger, more intelligent and faster in groups. But can such swarm intelligence also be transferred to quantum networks? A team led by Tanjung Krisnanda from Nanyang Technological University in Singapore asked themselves this question and found out: Yes, several poorly trained quantum networks together are more powerful than a single well-trained one. Their results are presented in the current issue of the scientific journal "Physical Review Applied".
In the social sciences, swarm intelligence is understood to mean that a decision made collectively by several people or a jointly achieved result is superior to the performance of a single expert. The principle is used in economic forecasting, public policy decision-making, medical diagnostics and scientific advice. The so-called "ensemble learning" in classical computer science is based on this. The idea is that a combination of different learning algorithms can be better than one algorithm alone.
When artificial neural networks contain quantum systems as nodes, they are referred to as neural quantum networks. In this case, the researchers used a special quantum reservoir network (QRN) that utilises the non-linear nature of quantum mechanical interactions. Each input influences the next reaction.
The input consists of two qubits that interact with the QRN for a certain period of time, so that the information about the input flows into the state of the QRN. By reading out the QRN nodes and processing the result using a trained output layer, the scientists are able to fully reconstruct the input state (tomography) or recognise whether the input qubits are entangled. "Assuming that the sum of the training runs is the same in both cases, we show that the collective result of the swarm estimates the input states better than the expert," the authors state. This conclusion also applies to the task of recognising entanglement.
In order to be able to compare experts and collectives, the scientists had imposed on themselves that the total number of training runs must be identical for both systems. However, if this restriction is relaxed, they write, the increase in the size of the swarm even offers the possibility of reducing the error rate to an arbitrarily low level. This, in turn, is a crucial prerequisite for being able to use machine learning reliably in real-life applications. "We assume that our results can be generalised to many typical quantum architectures and tasks of machine learning with quanta."
Spectrum of Science
We are partners of Spektrum der Wissenschaft and want to make well-founded information more accessible to you. Follow Spektrum der Wissenschaft if you like the articles.
[[small:]]
Image: Shutterstock

Experts from science and research report on the latest findings in their fields – competent, authentic and comprehensible.